CMIX
cmix is a lossless data compression program aimed at optimizing compression ratio at the cost of high CPU/memory usage. It gets state of the art results on several compression benchmarks. cmix is free software distributed under the GNU General Public License.
cmix works in Linux, Windows, and Mac OS X. At least 32GB of RAM is recommended to run cmix. Feel free to contact me at byron@byronknoll.com if you have any questions.
GitHub repository: https://github.com/byronknoll/cmix
Downloads
Source Code | Release Date | Windows Executable |
---|---|---|
cmix-v21.zip | September 10, 2024 | cmix-v21-windows.zip |
cmix-v20.zip | November 4, 2023 | cmix-v20-windows.zip |
cmix-v19.zip | August 29, 2021 | cmix-v19-windows.zip |
cmix-v18.zip | August 1, 2019 | cmix-v18-windows.zip |
cmix-v17.zip | March 24, 2019 | cmix-v17-windows.zip |
cmix-v16.zip | October 3, 2018 | cmix-v16-windows.zip |
cmix-v15.zip | May 5, 2018 | cmix-v15-windows.zip |
cmix-v14.zip | October 20, 2017 | cmix-v14-windows.zip |
cmix-v13.zip | April 24, 2017 | cmix-v13-windows.zip |
cmix-v12.zip | November 7, 2016 | cmix-v12-windows.zip |
cmix-v11.zip | July 3, 2016 | cmix-v11-windows.zip |
cmix-v10.zip | May 30, 2016 | cmix-v10-windows.zip |
cmix-v9.zip | April 8, 2016 | cmix-v9-windows.zip |
cmix-v8.zip | November 10, 2015 | |
cmix-v7.zip | February 4, 2015 | |
cmix-v6.zip | September 2, 2014 | |
cmix-v5.zip | August 13, 2014 | |
cmix-v4.zip | July 23, 2014 | |
cmix-v3.zip | June 27, 2014 | |
cmix-v2.zip | May 29, 2014 | |
cmix-v1.zip | April 13, 2014 |
Benchmarks
Corpus | Original size (bytes) | Compressed size (bytes) | Compression time (seconds) | Memory usage (KiB) |
---|---|---|---|---|
calgary.tar | 3152896 | 534512 | 2756.94 | 19359256 |
silesia | 211938580 | 28261094 | ||
enwik6 | 1000000 | 173431 | 831.74 | 19116288 |
enwik8 | 100000000 | 14623723 | 65154.91 | 20987016 |
enwik9 | 1000000000 | 107963380 | 622949.72 | 30950696 |
Compression and decompression time are symmetric. The compressed size can vary slightly depending on the compiler settings used to build the executable.
External Benchmarks
- Large Text Compression Benchmark
- Silesia Open Source Compression Benchmark
- Lossless Photo Compression Benchmark
Silesia Open Source Compression Benchmark
File | Original size (bytes) | Compressed size (bytes) |
---|---|---|
dickens | 10192446 | 1802071 |
mozilla | 51220480 | 6634210 |
mr | 9970564 | 1828423 |
nci | 33553445 | 781325 |
ooffice | 6152192 | 1221977 |
osdb | 10085684 | 1963597 |
reymont | 6627202 | 704817 |
samba | 21606400 | 1588875 |
sao | 7251944 | 3726502 |
webster | 41458703 | 4271915 |
xml | 5345280 | 233696 |
x-ray | 8474240 | 3503686 |
Calgary Corpus
File | Original size (bytes) | Compressed size (bytes) |
---|---|---|
BIB | 111261 | 17180 |
BOOK1 | 768771 | 173709 |
BOOK2 | 610856 | 105918 |
GEO | 102400 | 42760 |
NEWS | 377109 | 76389 |
OBJ1 | 21504 | 7053 |
OBJ2 | 246814 | 40139 |
PAPER1 | 53161 | 10831 |
PAPER2 | 82199 | 17169 |
PIC | 513216 | 21883 |
PROGC | 39611 | 8193 |
PROGL | 71646 | 8788 |
PROGP | 49379 | 6126 |
TRANS | 93695 | 9990 |
Canterbury Corpus
File | Original size (bytes) | Compressed size (bytes) |
---|---|---|
alice29.txt | 152089 | 31076 |
asyoulik.txt | 125179 | 29434 |
cp.html | 24603 | 4746 |
fields.c | 11150 | 1909 |
grammar.lsp | 3721 | 771 |
kennedy.xls | 1029744 | 7955 |
lcet10.txt | 426754 | 73365 |
plrabn12.txt | 481861 | 112263 |
ptt5 | 513216 | 21883 |
sum | 38240 | 6870 |
xargs.1 | 4227 | 1123 |
Description
I started working on cmix in December 2013. Most of the ideas I implemented came from the book Data Compression Explained by Matt Mahoney.
cmix uses three main components:
- Preprocessing
- Model prediction
- Context mixing
The preprocessing stage transforms the input data into a form which is more easily compressible. This data is then compressed using a single pass, one bit at a time. cmix generates a probabilistic prediction for each bit and the probability is encoded using arithmetic coding.
cmix uses an ensemble of independent models to predict the probability of each bit in the input stream. The model predictions are combined into a single probability using a context mixing algorithm. The output of the context mixer is refined using an algorithm called secondary symbol estimation (SSE).
Architecture
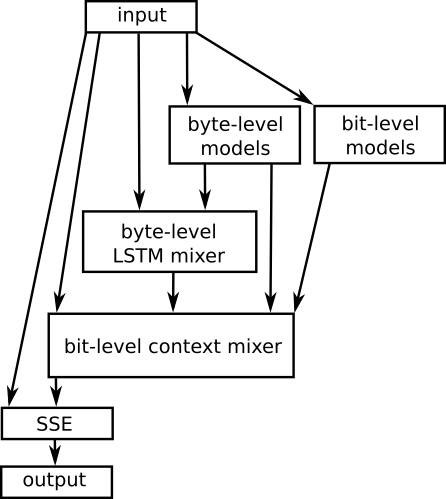
Preprocessing
cmix uses a transformation on three types of data:
- Binary executables
- Natural language text
- Images
The preprocessor uses separate components for detecting the type of data and actually doing the transformation.
For images and binary executables, I used code for detection and transformation from the open source paq8pxd program.
I wrote my own code for detecting and transforming natural language text. It uses an English dictionary and a word replacing transform. The dictionary comes from the fx-cmix Hutter prize entry and is 411,996 bytes.
As seen on the Silesia benchmark, additional preprocessing using the precomp program can improve cmix compression on some files.
Model Prediction
cmix v21 uses a total of 2,077 independent models. There are a variety of different types of models, some specialized for certain types of data such as text, executables, or images. For each bit of input data, each model outputs a single floating point number, representing the probability that the next bit of data will be a 1. The majority of the models come from other open source compression programs: paq8l, paq8pxd, and fxcm.
LSTM Mixer
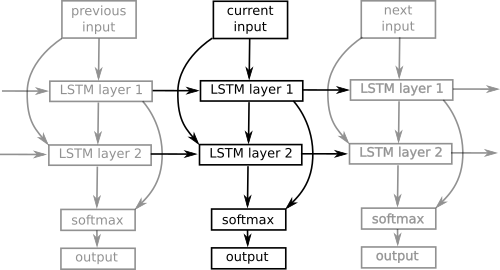
The byte-level mixer uses long short-term memory (LSTM) trained using backpropagation through time. It uses Adam optimization with layer normalization and learning rate decay. The LSTM forget and input gates are coupled. I created two other projects which compress data using only LSTM: lstm-compress and tensorflow-compress. Their results are posted on the Large Text Compression Benchmark.
Context Mixing
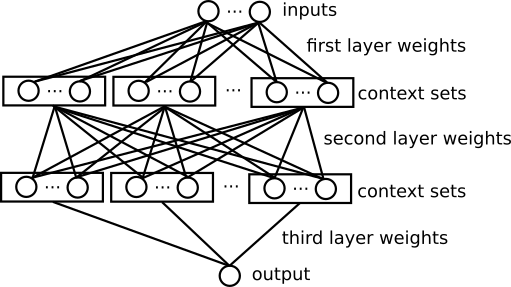
cmix uses a similar neural network architecture to paq8. This architecture is also known as a gated linear network. cmix uses three layers of weights.
- Loss function: cross entropy + L2 regularizer
- Activation function: logistic
- Optimization procedure: stochastic gradient descent
- Every neuron in the network directly tries to minimize cross entropy, so there is no backpropagation of gradients between layers.
- The inputs to each neuron (values between 0 to 1) are transformed using the logit function.
- Only a small subset of neurons are activated for each prediction. The activations are based on manually defined contexts (i.e. functions of the recent input history). One neuron is activated for each context. The context-dependent activations improve prediction and reduce computational complexity.
- Instead of using a global learning rate, each context set has its own learning rate parameter. There is also learning rate decay.
Comparison to PAQ8
cmix shares many similarities to the PAQ8 family of compression programs. There are many different branches of PAQ8. Here are some of the major differences between cmix and other PAQ8 variants:- In terms of performance, cmix typically has a better compression rate but is slower and uses more memory. cmix uses more predictive models than most PAQ8 variants.
- cmix uses a larger context mixing network. There are also several implementation details which differ (e.g. floating point arithmetic, learning rate decay, number of layers, etc).
- cmix uses two separate PAQ8 branches as internal models. One of the branches is fxcm. The other branch is a hybrid of several PAQ8 programs (i.e. a custom version of PAQ8 unique to cmix).
- The LSTM component is unique to cmix.
- The use of mod_ppmd. This is a PPM implementation that produces byte-level predictions. The predictions are used as input to the LSTM.
Acknowledgements
Thanks to AI Grant for funding cmix.
cmix uses ideas and source code from many people in the data compression community. Here are some of the major contributors:
- Matt Mahoney
- Alex Rhatushnyak
- Eugene Shelwien
- Márcio Pais
- Kaido Orav
- Mathieu Chartier
- Fabrice Bellard
- Artemiy Margaritov